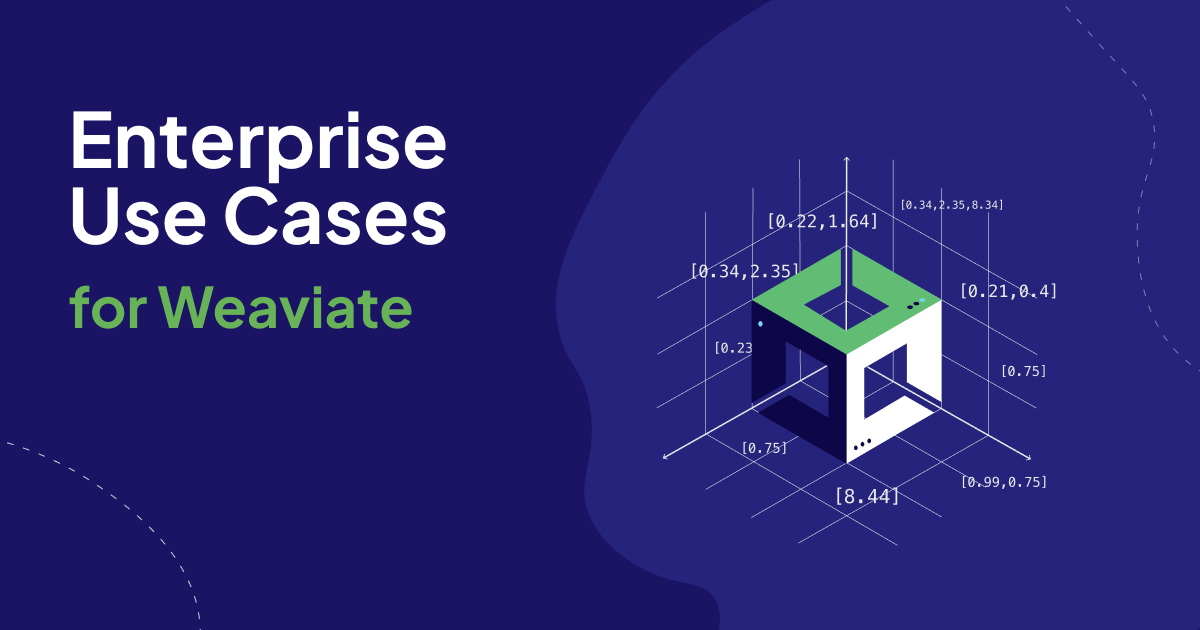
Enterprise Use Cases of Weaviate Vector Database
Explore enterprise use cases heavily used by our customers adopting generative AI features, search capabilities, and RAG with Weaviate vector database.
Explore enterprise use cases heavily used by our customers adopting generative AI features, search capabilities, and RAG with Weaviate vector database.
Verba is an open source Retrieval Augmented Generation (RAG) application built using a modular, customizable architecture that makes it easy for anyone to use AI methods to get personalized answers on their own data.
Weaviate 1.24 is released! Updates include named vectors, server telemetry, backend improvements, performance enhancements.
Learn how gRPC improves import and query speeds in Weaviate
Learn how to fine-tune Cohere's reranker and generate synthetic data using DSPy!
An end-to-end generative feedback loop demo using Weaviate, Ollama, Mistral and Snowflake’s Snowpark Container Services!
The new (v4) release of the Weaviate Python Client is - faster (gRPC), provides better IDE support and more type-safety, and many other developer experience improvements. Check out the new release and let us know what you think!
What you need to consider when using the Weaviate vector database with non-English languages, such as Hindi, Chinese, or Japanese.
We look at how to build Multimodal applications in TypeScript and dive into everything that needs to happen in between.
Weaviate 1.23 released with AutoPQ, flat indexing + Binary Quantization, OSS LLM support through Anyscale, and more!
A picture is worth a thousand words, so why just stop at retrieving textual context!? Learn how to perform multimodal RAG!