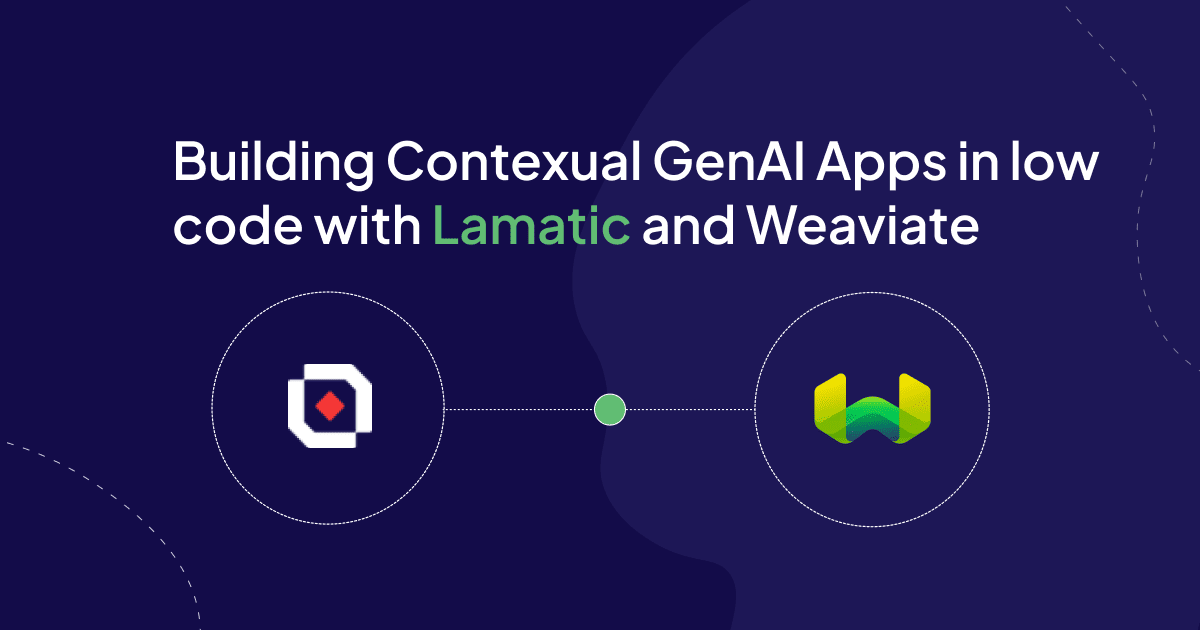
Build Contextual GenAI Apps in low code with Lamatic and Weaviate
Learn about Retrieval Augmented Generation (RAG), including architecture, use cases, implementation, and evaluation.
Learn about Retrieval Augmented Generation (RAG), including architecture, use cases, implementation, and evaluation.
1.27 adds filtered search and multi-target vector improvements, Jina V3 embedding support and more!
Learn about Retrieval Augmented Generation (RAG), including architecture, use cases, implementation, and evaluation.
Dive into how AI enables better eCommerce experiences with a focus on one critical component; Search.
Weaviate is happy to announce our inclusion in the Will Reed's list!
Learn about Late Chunking and how it may be the right fit for balancing cost and performance in your long context retrieval applications
Demo on how to ingest PDFs into Weaviate using Aryn.
Here's a recap of our Chicago Roadshow!
Add AI Capabilities to your Zoom Workplace and chat with your data in Zoom Team Chat
Learn about the power of generics and typing systems in Python and how they can improve your codebase.